Advancing AI-Driven Qualitative Analysis in Asia: A Case Study
- Craig Griffin
- Nov 5, 2024
- 6 min read
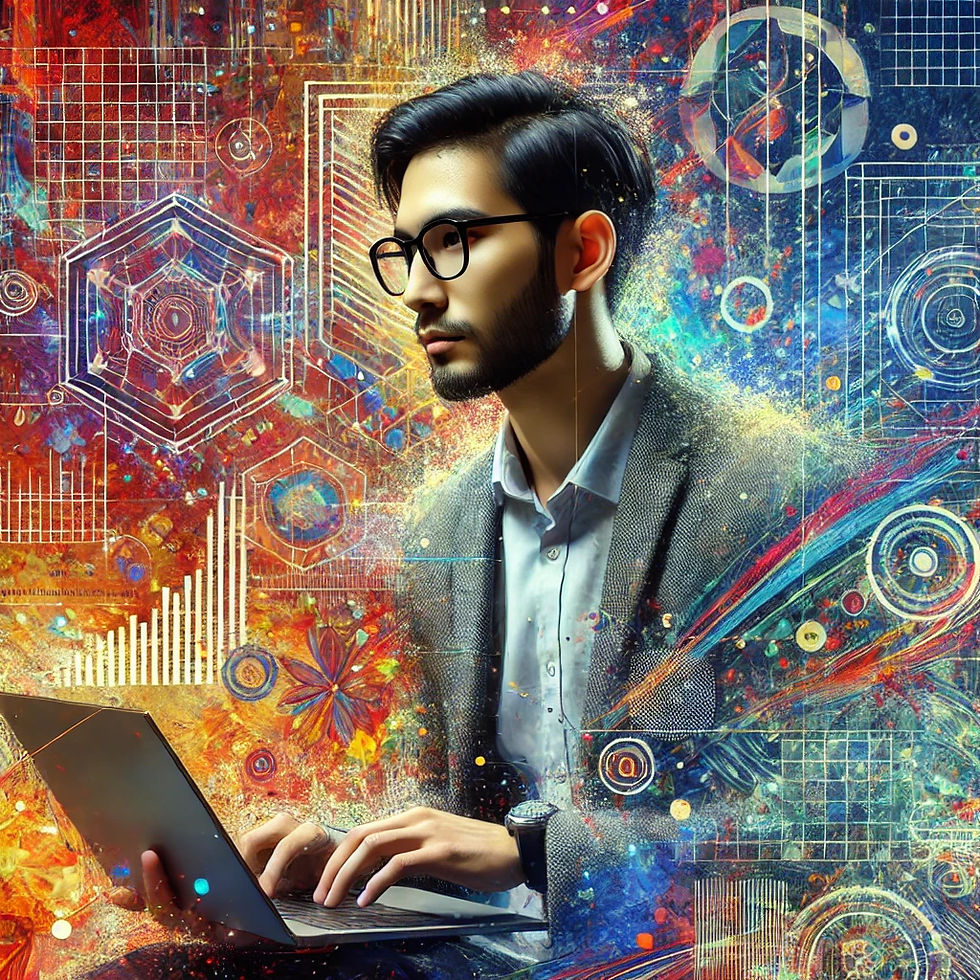
The potential of AI in qualitative research is transformative, promising faster insights, scalability, and innovative new approaches to data collection, analysis, and reporting. As the pace of AI transformation accelerates, research platforms are making notable strides in improving language and analysis support for diverse regions. However, when it comes to application in Asian markets, the journey toward realising AI's potential is complex. At FUEL Asia, we recognised an industry-wide need for rigorous, experience-based validation of AI tools to assess their true potential for non-Western languages and cultural contexts. This case study is part of that journey, where we put AI-driven qualitative research methods to the test using real-world data in the Thai language, measuring each approach’s strengths, limitations, and overall effectiveness.
As a sister article to The Democratisation of Research Innovation: How AI is Reshaping Qualitative Researchthis piece explores how different research technologies perform when faced with the nuances of Asian languages. It provides a practical, science-backed look at three main approaches: traditional human-only analysis, commercially available qualitative research platforms, and a DIY AI approach using generic AI tools not specifically designed for market research. Our aim is to shed light on how these methods stack up and to underscore the unique considerations and potential solutions for using AI tools to conduct qualitative research in Asia.
Experiment Overview: Three Approaches to Qualitative Analysis
This case study explores three approaches to qualitative analysis on a dataset of 10 online in-depth interviews conducted in Thailand. Each method offers distinct benefits and challenges, allowing us to test their limits for regional adaptability and effectiveness.
Human-Only Analysis: As our baseline, we utilised the skills of an experienced qualitative researcher to interpret the data manually. This traditional approach remains the gold standard for nuanced insights, setting the bar for quality.
Commercial Qualitative Research Tech Platforms: These specialised platforms are specifically designed to handle qualitative data at scale. In this case study, we tested 3 platforms. They offer user-friendly interfaces and often claim compatibility with diverse languages, allowing for streamlined analysis, quick deployment, and transparency in sourcing findings. However, as we’ll discuss, these platforms appear to show development lags in supporting some Asian languages.
‘DIY AI’: For our third approach, we adopted a more flexible DIY AI strategy, combining AWS for transcription, ChatGPT 4o for analysis and initial reporting and Gamma.app for creating a more visual PowerPoint report. While neither tool was designed explicitly for qualitative research, this setup allowed us to design an end-to-end solution for the entire analysis and reporting process.
Human-Only Analysis
Our researcher watched the interviews live and took comprehensive notes, allowing the researcher to capture subtle cues and contextual nuances in real time. Using a blend of thematic and narrative analysis, the researcher systematically identified recurring themes while also weaving in the narrative flow of each interview. This approach set the bar for quality, providing a rich, detailed understanding of the data and serving as a valuable benchmark for comparing the AI-driven methods.
Commercial Qualitative Research Tech Platforms
Commercial qualitative research platforms are designed specifically for streamlined, high-quality analysis. These tools already deliver on promises of ease and scalability, helping us generate insights faster than ever. Although some challenges remain in supporting Asian languages, we view these platforms as essential and believe they will continue to improve as demand in the region grows.
User-Friendly Design: These platforms are made for quick deployment and typically require little to no training. They’re intuitive, built to guide users through analysis with minimal friction, making them an appealing choice for fast-paced projects.
Transparency and Segment Analysis: Unlike some DIY approaches, commercial platforms allow users to trace findings back to their sources, increasing transparency and giving users confidence in their analysis. They also simplify segment-specific analysis and streamline the production of supporting media, such as video reels, which are often essential for stakeholder engagement.
Language Development Gaps: However, when it comes to the Thai language, these platforms reveal a notable development lag. Different platforms presented different problems; one platform could not recognize Thai in Zoom audio files, though it claimed to support the language. Another platform required us to split the analysis into two parts (due to token limits of the platform), which meant we had to create two reports and later combine them manually (using ChatGPT as a workaround).
Given the current pace of change, AI tools are all, now, a ‘work in progress’ and current commercial offerings may work better in some contexts than others. This will surely change quickly with ongoing development, fuelled by increasing demand from our region. Once language issues are addressed, they will offer an accessible and user-friendly option, especially for researchers with limited technical resources or time or willingness to experiment.
‘DIY AI’ Approach: Flexibility, Customisation, and Price
The flexibility of a DIY AI approach was clear from the outset. With the three main tools we used, we created workflows that were highly customised to our project needs, including the desire to maintain a consistent brand identity and style. These workflows can be easily adapted to different projects or client needs. The customisation potential here was unparalleled, allowing us to experiment with various qualitative analysis approaches rapidly and iteratively create something we were proud to put our brand behind.
While this flexibility offers an edge, it does come with an initial time investment. Unlike specialised platforms, setting up DIY AI requires initial adjustments to workflows and prompts, along with some trial and error to get the best results. However, this flexibility can be a major advantage, allowing us to quickly adapt analysis and reporting to meet the unique needs of each project. Aside from time costs, this approach is inexpensive – a Chat GPT subscription and about USD 15 of transcription costs.
Our results show that, when designed well, DIY AI can match - and sometimes even outperform - commercial platforms. With carefully crafted prompts and outputs aligned to FUEL Asia’s standards, we retained strong control over analysis quality and reporting. This adaptability is crucial in producing tailored, impactful, and powerfully delivered insights.
Comparing the Three Approaches: Key Learnings for Asian Markets
When evaluating our findings across the three approaches, several key findings emerged, highlighting both the challenges and opportunities in deploying AI-driven qualitative research for Asian markets.
DIY AI as a Solution to Language and Localisation Gaps: In our experience, DIY AI emerges as a viable and powerful solution to overcome current limitations in language support. This flexible, high-control approach allows researchers to achieve depth without the restrictions of current platform limitations, proving that AI as a co-pilot can be transformative for qualitative research when tailored precisely. The potential for ‘better’ qualitative research using AI as a co-pilot is already here.
Addressing Development Gaps in Asia: The unique requirements of Asian markets require greater attention from platform developers. While commercially available platforms offer ready-made solutions, they seem to work better with some languages than others, making the DIY approach an attractive alternative. For qualitative researchers working in Asian markets, DIY AI offers greater control over language handling and analysis and reporting approaches, facilitating deeper insights without relying solely on tools that may not yet fully support non-Western languages.
Localised Pricing for Market Penetration: FUEL Asia believes that localised pricing, along with culturally attuned development, will be critical in driving platform adoption in Asia, ultimately benefiting the global industry with more inclusive AI tools. The cost for producing human transcripts and doing human analysis is relatively low in some countries we work in, and commercial tools need to be priced accordingly.
The Road Ahead: Inviting the Industry to Bridge the Gap
As our case study demonstrates, AI is a powerful enabler of qualitative research, with the potential to enhance analysis speed, consistency, and adaptability. Yet, from our initial small scale trial, there appears to be much to be done to bridge the development gap in Asian markets, where language and budget considerations present distinct challenges. To advance the conversation and explore new solutions, FUEL Asia has partnered with Insight Platforms to co-host the upcoming Asia Digital Insights Summit in January, a landmark event for the research community in Asia, that brings together leaders in research and technology to discuss the future of technology-driven insights in Asia.
The summit will feature a dynamic agenda, including keynote sessions, expert panels, and live demos of breakthrough research technologies – addressing both quantitative and qualitative use cases.
Conclusion: Collaborating for an AI-Driven Future in Asian Research
This case study illustrates the evolving landscape of AI in qualitative research, where both tailored DIY approaches and specialised platforms have a role to play. FUEL Asia remains committed to advancing this dialogue, and we invite you to join us at the Asia Digital Insights Summit. Together, we can drive research innovations that strengthen the industry, creating solutions that meet the diverse and evolving needs of Asian markets.
Comments